Generative AI, a fascinating subset of Artificial Intelligence that’s shaping the future right before our eyes. But what exactly is Generative AI?
Generative AI is about machines creating new, original content. From images and music to text and beyond, it leverages vast data to produce work that resonates with human creativity.
Why does this matter? Generative AI opens doors to innovation we’ve only just begun to explore. It’s not about replacing human effort; instead, it amplifies our creative potential, making the leap from imagination to reality quicker and more efficient.
Generative AI Vs Traditional AI Models
So, how does Generative AI stand apart from the traditional AI we’ve come to know? Traditional AI excels in organizing and analyzing data, making predictions, and identifying patterns. It’s the technology that helps us make sense of the vast information landscape.
Generative AI, however, takes a bold step forward. It doesn’t just further analyze complex data; it creates. It uses its understanding of data to produce entirely new pieces that mirror human creativity. This isn’t about asking what exists in the data; it’s about asking what could exist.
This distinction is powered by recent breakthroughs in AI, particularly in deep learning and neural networks. Tools like generative adversarial networks (GANs) and large language models have become the architects of this new creative frontier, offering not just answers but inspiration.
Generative AI’s application spectrum is vast, from streamlining design processes to inventing novel solutions in healthcare. Its potential is as broad as the human imagination, promising to revolutionize how we approach problems and conceive solutions.
What are Generative AI Models?
Generative AI models stand at the forefront of technological innovation, transforming how we approach data to create new content.
Here, we’ll embark on a journey through the core architectures that power this innovative field. So, buckle up and get ready for a ride through the intricate yet captivating landscape of generative models.
1. Generative Adversarial Networks (GANs) and Their Impact
Generative Adversarial Network, or GANs, introduce a dynamic duo of neural networks: the Generator and the Discriminator.
The Generator’s job is to create data mimicking the real datasets it’s trained on, while the Discriminator evaluates this data for authenticity.
Their interplay enhances the Generator’s output over time, leading to highly realistic results. GANs have made significant strides in fields like image generation, contributing to advancements in virtual reality and digital art.
2. The Role of Variational Autoencoders (VAEs)
Variational Autoencoders, known as VAEs, operate on a principle of encoding and decoding.
They compress data into a condensed form and then reconstruct it, learning to capture the essence of the input data.
This process allows VAEs to generate new data points with similar characteristics to the training set. Applications range from creating diverse images to designing complex 3D models, showcasing their versatility in generative tasks.
3. Autoregressive Models in AI
Autoregressive models take a sequential approach to data generation, where each new output depends on the preceding ones. This method is particularly effective in text and speech generation, where coherence and context are crucial.
By analyzing patterns in the sequence of data, autoregressive models can predict subsequent elements, making them invaluable in language models and time-series forecasting.
4. Transformer Model in Generative AI
Transformers have revolutionised the use of AI in understanding and generating human language. Unlike traditional models that process data in order,
Transformers model can look at an entire sequence simultaneously, allowing for more nuanced understanding and generation of text.
This has led to breakthroughs in machine translation, content creation, and even in generating code, significantly expanding the scope of generative AI applications.
Each of these architectures plays a critical role in the expanding landscape of generative AI. From the competitive learning environment of GANs to the efficient data encoding-decoding of VAEs, the sequential logic of autoregressive models, and the contextual comprehension of Transformers, generative AI models are reshaping our digital world.
Also Read: What is Generative AI: A Complete Overview of Working, Benefits and Challenges
Types of Generative AI Models
Exploring generative AI models unveils a realm where innovation meets practicality, creating solutions that push the boundaries of what’s possible with technology.
1. Foundation Models
Foundation models stand as the pillars of modern AI, offering a robust starting point for various machine learning endeavors.
These models, including well-known examples like BERT and GPT-4, are versatile powerhouses capable of tasks ranging from natural language processing to intricate image analysis.
Their adaptability and broad applicability make them a cornerstone in the development of new AI applications, streamlining the process and enhancing efficiency.
2. Diffusion Model
Diffusion model introduces a novel approach to data generation, akin to an artist gradually refining a painting. Starting with a canvas of noise, these models meticulously learn to reverse this process, gradually revealing detailed and coherent outputs.
This technique has revolutionized image generation, offering new methods for artistic creation, photo enhancement, and even contributing to advances in scientific visualization.
3. Neural Network Models
At the core of many AI systems are neural network models, inspired by the neural architecture of the human brain.
These models, including recurrent neural networks (RNNs) and convolutional neural networks (CNNs), are adept at processing sequential data and analyzing visual content, respectively.
Their structure and functionality make them invaluable across a wide array of applications, from language translation to visual recognition tasks.
4. Machine Learning Models
Machine learning models are the engines driving AI’s predictive and decision-making capabilities. They range from supervised learning models, which learn from labeled datasets to reinforcement learning models, which improve through trial and error.
These models are instrumental in personalizing digital experiences, optimizing business processes, and even guiding autonomous systems.
By integrating these generative AI models into their operations, businesses and creators can unlock new levels of efficiency, creativity, and innovation.
Whether it’s enhancing customer interactions, streamlining product development, or exploring new artistic frontiers, generative AI holds the key to a myriad of possibilities.
The Power of Generative AI in Various Domains
Generative AI is revolutionizing industries far and wide, transforming the way we create, analyze, and personalize.
Through these examples, it’s clear that generative AI is not just a technological tool but a catalyst for innovation across various domains. By unlocking new creative potentials, enhancing personalization, and solving complex problems, generative AI is setting the stage for a future where technology and human creativity converge in unprecedented ways.
Let’s explore ai research and the incredible impact of generative AI across various sectors.
1. Generative AI in Art and Design
In the world of art and design, generative artificial intelligence acts as a muse and collaborator, enabling artists to push the boundaries of creativity. Tools like DeepArt leverage convolutional neural networks to transform basic sketches into stunning visuals, opening up new avenues for artistic expression.
These AI-driven platforms are not just tools but partners in the creative process, enhancing human creativity with their vast computational power and ability to recognize and replicate complex patterns.
2. Text Generation and Large Language Models
Generative AI has also made significant strides in content creation, particularly through large language models like GPT-3.
These models can produce human-like text based on input, covering a wide array of applications from writing assistance to programming help.
The ability of these models to understand and generate contextually relevant content has made them invaluable assets in content generation, making the creation process more efficient and diverse.
3. The Use of Generative AI in Game Development
In game development, generative AI is transforming the narrative and environmental design, offering dynamic and immersive experiences.
AI Dungeon, a text-based adventure game powered by GPT-3, exemplifies this by generating unique storylines based on player input, ensuring no two gaming experiences are the same.
This adaptability and creativity make generative AI a game-changer in developing engaging and personalized gaming worlds.
4. How Generative AI Contributes to Data Augmentation and Synthetic Data
Data is the lifeblood of the digital age, and generative AI plays a crucial role in data augmentation and the creation of synthetic data.
By generating realistic datasets, AI models help in training machine learning algorithms where real data might be scarce or sensitive.
This capability is vital in sectors like healthcare, where synthetic data can be used for research without compromising patient privacy.
5. Generative AI in User Personalization
Generative AI is at the forefront of personalizing user experiences, from tailored recommendations in e-commerce to customized content in digital media.
By analyzing individual preferences and behaviors, AI systems can generate personalized content, enhancing user engagement and satisfaction.
This level of personalization is reshaping consumer expectations and setting new standards for digital interactions.
6. Transforming Healthcare with Generative AI Applications
Perhaps most profoundly, generative AI is making waves in healthcare, from drug discovery to personalized treatment plans.
By analyzing vast datasets, AI models can uncover new drug compounds, predict their efficacy, and tailor treatments to individual genetic profiles.
This not only accelerates the drug development process but also paves the way for more personalized and effective healthcare solutions.
Generative AI’s versatility and innovative capabilities are ushering in a new era across various domains.
By enhancing creativity, streamlining content generation, revolutionizing game development, enriching enterprise data mine, personalizing user experiences, and transforming healthcare, generative AI is not just a technological advancement but a catalyst for cross-industry innovation.
Also Read: How Generative Artificial Intelligence Drives Compliance and Innovation: A
Comprehensive Overview
Navigating the Challenges of Generative AI
Exploring generative AI is akin to navigating a complex maze; it’s filled with potential but also presents significant challenges that demand attention.
Understanding the Limitations and Challenges of Generative Models
The power of generative AI models is undeniable, yet they come with their own set of limitations. A key challenge is their heavy reliance on data quality.
In essence, if the training data is biased or incomplete, the AI’s output will likely reflect these flaws. Furthermore, the computational demands for training these models are substantial, requiring not just powerful hardware but also efficient algorithms to make the process feasible.
Ethical Considerations and the Quality of AI-Generated Content
Ethics take center stage when discussing generative AI. The technology’s ability to produce content that closely mimics human output brings forth significant ethical dilemmas.
For instance, the creation of deepfakes raises concerns about consent, identity, and the potential for spreading misinformation. Ensuring that AI-generated content is used responsibly is paramount in maintaining trust in this evolving technology.
Delving into the Challenges in Generative AI
One of the trickier aspects of generative AI is controlling its output. Ensuring that the content generated aligns with user intentions requires a delicate balance between guiding the AI and allowing it the freedom to innovate.
This balance is crucial in maintaining the utility human intelligence and creativity of generative AI systems.
1. Addressing Mode Collapse and Its Mitigation
Mode collapse, where a generative model repetitively produces similar outputs, limits the diversity and utility of AI-generated content.
Tackling this issue involves refining the model’s architecture and introducing more varied training data to encourage a broader range of outputs.
2. Evaluating Generative Models: Inception Score and FID
Assessing the performance of generative models is critical in understanding their capabilities and limitations.
Metrics like the Inception Score and Frechet Inception Distance (FID) offer a way to quantify the diversity and realism of AI-generated content, providing valuable feedback for model improvement.
3. Tackling Data Bias and Ensuring Fairness in AI
Data bias remains a significant challenge, as it can lead to AI outputs that reinforce existing prejudices.
Addressing this issue involves a proactive approach to data collection and model training, ensuring that generative AI systems promote fairness and diversity.
In navigating the complexities of generative AI, we must be mindful of these challenges. By addressing them head-on with a blend of technical innovation and ethical consideration, we can harness the full potential of generative AI while minimizing its risks.
How can companies embrace generative ai models?
Embracing the era of generative AI is a strategic move for companies looking to stay ahead. It requires a solid foundation in digital technology, a commitment to innovation, and a clear vision for integrating AI into business operations.
1. Building a robust digital infrastructure is the first step. The computational power needed for generative AI demands cutting-edge, cloud-based solutions and a
comprehensive data platform. This setup supports the sophisticated demands of generative AI, allowing businesses to leverage its full potential.
2. Cultivating a culture that values innovation and learning is also essential. Generative AI represents a new way of solving problems and discovering opportunities. Companies that encourage a mindset of exploration and adaptability are more likely to
succeed in this new landscape.
3. Strategically incorporating generative AI into business processes is another key factor. Identifying where generative AI can make the most impact—whether in improving customer experiences, streamlining operations, or innovating products—is crucial. A strategic approach ensures that AI adoption aligns with broader business goals.
4. Lastly, ethical use of AI is important. Companies must establish guidelines for responsible AI use, ensuring that generative AI technologies are used ethically and safely. This not only protects the company and its customers but also contributes to the
responsible development of AI technologies.
Also read: Generative AI in Healthcare: A Guide to Revolutionising Patient Care
What are the Benefits of Generative AI?
The adoption of generative AI (GenAI) is transforming industries by offering solutions that significantly enhance efficiency, creativity, and personalization. Here’s how businesses are reaping the benefits of generative ai:
1. Faster Product Development: Generative AI models are transforming the product development landscape. By generating innovative ideas and concepts swiftly, it drastically cuts down the time traditionally needed for manual brainstorming and prototyping. This rapid pace allows businesses to bring new products to the market faster than ever before, staying ahead in competitive landscapes.
2. Enhanced Customer Experience: Personalization is where Generative AI system truly shines. It tailors content, recommendations, and interactions to individual preferences, making every customer feel valued.
Moreover, chatbots powered by GenAI understand context better, leading to more natural conversations and improved customer service. Furthermore, AI models empower chatbots, making customer service interactions more intuitive and contextually relevant.
3. Improved Employee Productivity: By taking over routine tasks, generative AI allows employees to concentrate on strategic and creative work.
GenAI is a powerful ally in the workplace, automating routine tasks such as content creation and data analysis. This frees up employees to focus on more complex and creative tasks, boosting overall productivity and job satisfaction.
4. Creative Exploration: Generative models offer a sandbox for creativity, enabling professionals in creative fields to explore novel ideas and concepts.
For creatives, Generative AI model opens up a world of possibilities. It encourages experimentation by providing a vast array of options in art, writing, and design that were previously unattainable, pushing the boundaries of human creativity.
5. Content Generation: The capacity of generative AI technology to produce diverse content, from articles to visuals, is particularly beneficial for marketing and storytelling From marketing materials to social media posts, generative artificial intelligence can produce a wide variety of content quickly and efficiently.
This not only aids in keeping content fresh and engaging but also supports storytelling in unique and innovative ways.
6. Data Augmentation: In the realm of machine learning, generative artificial intelligence model is invaluable for generating additional training data, enhancing the accuracy and generalization of models.
Generative AI plays a crucial role in data augmentation, enriching datasets to train machine learning models more effectively. This improves the models’ accuracy and their ability to generalize across different scenarios.
7. Research and Exploration: Generative AI accelerates research by enabling the simulation of data, testing hypotheses, and exploring scenarios that would otherwise require significant time and resources.
This capability is groundbreaking, especially in fields requiring extensive data analysis and hypothesis testing.
Embracing Generative AI allows companies to leverage these benefits, leading to enhanced creativity, improved productivity, and more personalized customer experiences.
Future Directions in Generative AI
The journey of generative AI is unfolding new paths, promising a future where technology and human creativity intersect like never before. Here’s a glimpse into what lies ahead.
1. Pioneering Few-Shot and Zero-Shot Learning
In the world of AI, the ability to learn from limited examples is revolutionary. Few-shot and zero-shot learning are setting the stage for AI models that require minimal data to understand new concepts. This breakthrough could drastically reduce the time and resources needed for training AI, making it more adaptable and efficient.
2. Interpretable Generative Models
Transparency in AI’s decision-making is becoming increasingly important. Efforts to develop interpretable generative models aim to make AI’s thought processes clearer, fostering trust, especially in critical applications where understanding AI’s logic is essential.
3. AI Decision-Making Processes
Demystifying how AI arrives at its conclusions is crucial for its wider acceptance. Initiatives to illuminate AI’s decision-making will bolster confidence in its applications, ensuring its decisions are both reliable and comprehensible.
4. The Rise of Hybrid Models
The fusion of different AI techniques into hybrid models is a frontier of innovation. This blend harnesses the strengths of various methods, paving the way for more robust and versatile AI solutions.
5. Merging Creativity with Adaptability through AI Synergies
The synergy between generative AI and human creativity heralds a new era of innovation. This collaboration promises to break new ground, offering solutions that are both novel and effective.
As we navigate the evolving landscape of generative AI, its potential to drive progress and transformation across various domains becomes increasingly evident. By exploring these future directions, we can harness the full spectrum of possibilities that generative AI holds.
Enhancing Healthcare Communication with Voiceoc’s Generative AI
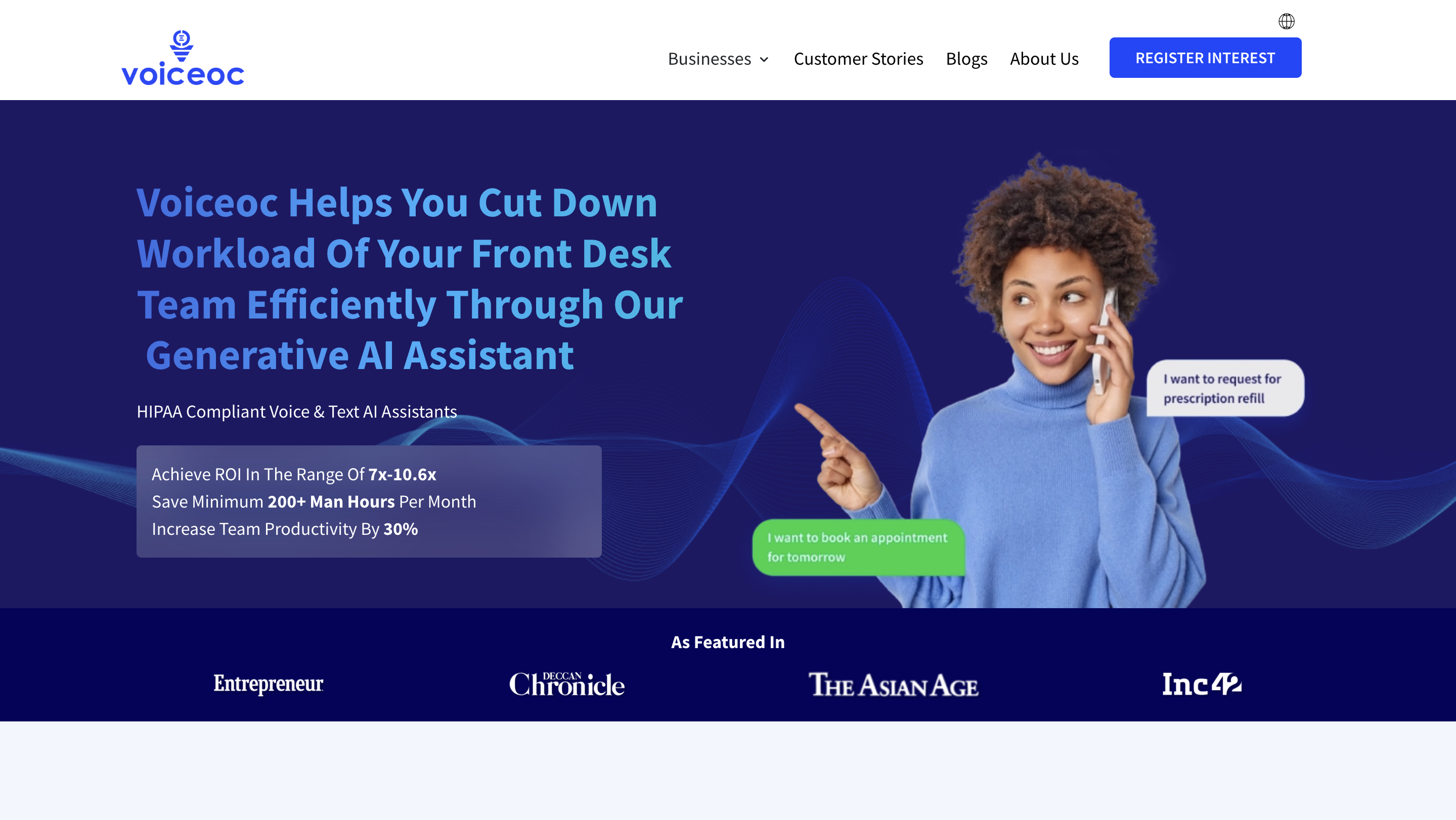
In the realm of healthcare, where every interaction holds significant value, Voiceoc is revolutionizing patient engagement through the power of conversational AI.
Leveraging a robust AI engine, nourished by over 1 million patient conversations monthly, Voiceoc stands at the forefront of transforming healthcare communication, aligning seamlessly with the advancements in generative AI.
1. Streamlining the Patient Journey: Voiceoc’s AI engine automates the entire spectrum of the patient journey, creating a seamless experience from initial inquiry to post-treatment communication.
This comprehensive automation includes symptom diagnosis, appointment booking, support services, accessing medical records, and follow-up interactions, ensuring every step is handled with precision and empathy.
2. Simplifying Appointment Management: With Voiceoc, managing appointment bookings becomes effortlessly efficient. The platform’s integration of NLP-driven intelligence ensures a free-flowing, contextual interaction with patients, akin to a human conversation.
This capability, combined with pre-trained AI models that seamlessly integrate with Health Information Systems (HIS) and Laboratory Information Systems (LIS), accelerates the deployment of Voiceoc’s solutions, enabling faster go-to-market strategies for healthcare providers.
3. Empowering Conversations with AI: Voiceoc’s AI engine is meticulously designed to handle nuanced patient communications, transferring chats to human agents only under specific conditions, such as negative feedback or explicit patient requests.
This intelligent system, supported by a proprietary queue mapping algorithm, significantly reduces drop-off rates, enhancing patient satisfaction.
4. Innovating with Auto-Suggestions and Analytics: Voiceoc takes patient interactions to the next level with AI-powered auto-suggestions, facilitating more meaningful and efficient conversations.
Additionally, the platform offers insightful analytics on agent performance, providing valuable feedback and areas for improvement.
Conclusion
Generative AI stands at the forefront of artificial intelligence, poised to revolutionize various industries with its creative potential and problem-solving capabilities.
Through the utilization of generative models and machine learning algorithms, Gen AI enables the automated generation of text, images, and even complex 3D models, offering unprecedented opportunities for innovation and efficiency.
The ability of Gen AI to automate end-to-end processes, manage appointment bookings seamlessly, and engage patients with human-like conversational experiences exemplifies its potential to reshape the future of healthcare and beyond.
With ongoing advancements in technology and an expanding ecosystem of AI-driven solutions, the journey towards unlocking the full potential of Gen AI promises to be both exciting and transformative.
In an era where generative AI is reshaping industries, Voiceoc embodies the synergy between technological innovation and human-centric care in healthcare communications. By bridging the gap with conversational AI, Voiceoc not only delights patients but also sets a new standard for patient engagement in the digital age.