Generative AI, a cornerstone of artificial intelligence, harnesses the power of deep-learning models to create diverse and high-quality content, spanning text, images, and beyond.
At its core, generative AI revolves around the concept of machines mimicking human creativity and intelligence, producing outputs that often blur the lines between human and machine-generated content.
Generative AI operates on the principle of neural networks, intricate systems inspired by the human brain.
These networks are trained on vast datasets, absorbing patterns and intricacies to generate new content autonomously. Through a process of trial and error, the models refine their outputs, striving for accuracy and coherence.
Exploring the Concept of Generative AI
In simpler terms, generative artificial intelligence acts as a virtual artist, capable of painting vivid landscapes with pixels and weaving intricate narratives with words.
Generative AI’s capabilities extend far beyond mere text and images. It ventures into realms like music composition, code generation, and even drug discovery.
With each iteration, these models push the boundaries of what’s possible, opening doors to new realms of innovation and creativity.
Generative AI, with its ability to understand and manipulate data, holds immense potential across various domains. From assisting in content creation to aiding in problem-solving, its applications are as diverse as they are impactful.
By harnessing the power of generative AI, businesses can streamline workflows, unlock new revenue streams, and gain a competitive edge in today’s dynamic market landscape.
Whether it’s generating personalised product recommendations or crafting compelling marketing materials, the possibilities are limitless.
In summary, generative artificial intelligence represents a paradigm shift in how we interact with technology. It’s not just about automating tasks; it’s about empowering creativity, sparking innovation, and reshaping the way we perceive artificial intelligence.
Key Components of Generative AI Systems
In our exploration of generative AI, one crucial aspect is the availability of comprehensive training data. Training data serves as the foundation upon which generative AI models are built.
1. Training Data: It encompasses vast amounts of information used to train these models to perform specific tasks, ranging from generating images to creating text. However, obtaining high-quality training data can be a challenging endeavor. It requires meticulous curation and preparation to ensure that the data accurately represents the desired outcomes.
2. Machine Learning: Machine learning techniques form the core of generative AI, enabling models to learn from data and generate new content autonomously
These techniques encompass a broad range of algorithms and methodologies, each tailored to specific tasks and objectives.
From supervised learning to unsupervised learning and reinforcement learning, machine learning techniques drive the development of generative AI models by providing the tools and frameworks necessary for training and optimisation.
Moreover, ongoing research in machine learning continues to push the boundaries of what is possible with generative AI, leading to the development of more advanced and sophisticated models capable of generating increasingly realistic and diverse outputs.
3. Language Models: Language models play a pivotal role in the realm of generative AI, serving as the backbone for text generation and natural language processing tasks. These models are trained on vast corpora of text data, enabling them to understand and generate human-like text.
Language models underpin a wide range of applications, from virtual assistants to automated content generation systems. Their ability to comprehend and produce coherent and contextually relevant text has revolutionised various sectors, including journalism, customer service, and entertainment.
Moreover, advancements in language models have led to the development of more sophisticated generative AI systems capable of generating highly realistic and contextually appropriate textual content across multiple languages and domains.
As such, machine learning serves as the cornerstone of generative AI, laying the groundwork for future innovations and breakthroughs in artificial intelligence. generative ai model, generative ai model, diffusion models, diffusion models, diffusion models, diffusion models.
How Does Generative AI Work?
Generative AI, an intricate subset of artificial intelligence, operates through sophisticated machine learning models trained on vast datasets. Let’s delve into the inner workings of generative AI to understand its mechanisms better.
The Inner Workings of Generative AI
At the heart of generative AI are foundation models (FMs), which serve as the backbone of the technology. These models undergo training on a diverse array of generalised and unlabelled data, enabling them to tackle a wide spectrum of tasks with ease. Leveraging learned patterns and relationships, FMs excel at predictive tasks, such as image generation and text completion.
For instance, in image generation, a foundation model analyzes the input image and produces a refined version with enhanced clarity and detail.
Similarly, in text generation, the model predicts the next word in a sequence based on the context and preceding words, employing probability distribution techniques for accurate predictions.
Deep Dive into Generative Models
Large language models (LLMs), a subtype of Foundation models, play a pivotal role in generative AI. Notably, OpenAI’s generative pre-trained transformer (GPT) models exemplify the capabilities of LLMs.
These models specialise in language-centric tasks, including summarisation, text generation, classification, conversational engagement, and information extraction.
What sets LLMs apart is their versatility in handling multiple tasks seamlessly. Empowered by billions of parameters, LLMs like GPT-3 possess the remarkable ability to generate content with minimal input.
Their extensive pertaining exposure to internet-scale data equips them with the knowledge to navigate diverse contexts effectively.
In summary, generative AI thrives on the synergy between foundation models and large language models, harnessing their collective intelligence to produce innovative outputs across various domains.
Evaluating Generative AI Models
Many Generative AI models represent the culmination of advancements in machine learning and artificial intelligence. These models leverage complex algorithms to generate new content, such as images, videos, and text, based on patterns learned from training data.
The development of generative AI models involves intricate processes that require expertise in machine learning and data science. By iteratively training on vast amounts of data, generative AI system gradually learn to mimic human creativity and intelligence, leading to the creation of highly realistic and innovative outputs.
In this section, we delve into the methodologies and considerations involved in evaluating generative AI models.
Criteria for Assessing Generative AI Models
When evaluating generative AI models, several key criteria come into play, each essential for determining the model’s efficacy and performance:
1. Quality: The quality of generated outputs is paramount, particularly in applications involving direct interaction with users. Whether it’s speech generation or image synthesis, high-quality outputs are imperative for seamless user experiences.
For instance, in speech generation, clear and understandable speech is essential, while in image synthesis, the generated images should closely resemble natural ones.
2. Diversity: A robust generative model should be capable of capturing the diverse modes present in its underlying data distribution. This diversity ensures that the generated outputs encompass a wide range of variations and do not exhibit biases towards specific attributes or patterns.
By maintaining diversity, generative models can produce outputs that are more representative of the underlying data distribution.
3. Speed: In many applications, particularly those involving real-time interactions or content generation workflows, the speed of generation is crucial.
Generative AI models should be able to produce outputs swiftly without compromising on quality or diversity.
Efficient generation processes enable seamless integration of generative AI into various applications, enhancing productivity and user satisfaction.
Metrics and Techniques for Evaluation
Evaluating generative AI models often involves the use of specific metrics and techniques tailored to assess their performance accurately. Some common evaluation metrics and techniques include:
1. Perceptual Quality Metrics: Metrics such as inception score and Fréchet inception distance (FID) are commonly used to assess the perceptual quality of generated images.
These metrics measure the similarity between generated images and real images based on various visual features.
2. Diversity Measures: Metrics like diversity score and entropy are employed to quantify the diversity of generated outputs.
These measures help ensure that generative models produce outputs that cover a wide range of variations and avoid generating similar or redundant samples.
3. Human Evaluation Studies: Conducting human evaluation studies where human assessors provide feedback on the quality, diversity, and overall performance of generative AI models can offer valuable insights.
Human evaluation helps validate the effectiveness of generative models from a user-centric perspective.
Practical Examples of Model Evaluation
Generative AI is built upon a foundation of diverse models that serve as the building blocks for innovation and creativity. These foundation models encompass a broad spectrum of architectures and techniques, each designed to address specific challenges and requirements.
From recurrent neural networks to transformer architectures, foundation models form the backbone of generative AI research and development.
By leveraging these foundational frameworks, researchers and practitioners can explore new frontiers in generative AI, pushing the boundaries of what is possible and unlocking new opportunities for innovation and discovery.
To illustrate the evaluation process, let’s consider practical examples of different types of generative AI models:
1. Diffusion Models: These models iteratively introduce controlled random changes to an initial data sample, gradually transforming it into a new sample. Evaluation involves assessing the coherence and realism of generated samples after the full diffusion model process.
2. Generative Adversarial Networks (GANs): GANs employ a competitive training process between a generator and a discriminator network. Evaluation focuses on measuring the realism of generated samples and the ability of the discriminator to differentiate between real and fake data.
3. Variational Auto encoders (VAEs): VAEs learn a latent representation of input data, and reconstruct input samples from this latent space. Evaluation entails assessing the fidelity of reconstructed samples compared to the original data and the diversity of generated samples from the latent space.
4. Transformer-Based Models: These models, such as GPT (Generative Pre-trained Transformer), excel in text generation tasks. Evaluation includes assessing the coherence, diversity, and relevance of generated text samples across various contexts and applications.
Also Read: How can AI Chatbots help enhance patient engagement
How to Develop Generative AI Models
Generative AI has revolutionised various industries, offering the capability to generate data, images, and even text autonomously.
In this section, we’ll explore the step-by-step process of developing generative AI models, including choosing the right frameworks and tools, and best practices to ensure optimal outcomes.
Step-by-Step Guide to Developing Generative AI Models
Developing generative AI models involves several intricate steps, each crucial for achieving desired results. Here’s a simplified breakdown:
1. Understanding Generative Models: Generative models, such as diffusion models,variational auto encoders (VAEs), and generative adversarial networks (GANs), form the backbone of generative AI. Each model offers unique capabilities and benefits.
2. Model Selection: Choose the appropriate generative model based on the specific requirements of your project. Consider factors like the type of data you’re working with, the level of detail needed in generated outputs, and the speed of generation.
3. Data Preparation: Prepare your training data meticulously. Clean, well-organized data is essential to create realistic data used for training accurate and effective generative models. Ensure your dataset is representative of the real-world scenarios you aim to replicate.
4. Model Training: Train your selected generative model using the prepared dataset. This step involves feeding the model with data and iteratively adjusting its parameters to minimize errors and improve performance.
5. Evaluation and Validation: Evaluate the trained model’s performance using appropriate metrics and validation techniques. Assess factors like output quality, diversity, and speed to ensure the model meets your expectations.
6. Fine-Tuning: Fine-tune the model based on evaluation results and feedback. Adjust parameters, optimize algorithms, or incorporate additional data to enhance model performance further.
7. Deployment: Once satisfied with the model’s performance, deploy it for real-world applications. Monitor its performance in production environments and make necessary adjustments as needed.
Choosing the Right Frameworks and Tools
Selecting the right frameworks and tools is critical for efficient and effective generative AI model development.
Consider factors like community support, scalability, and compatibility with your existing infrastructure. Popular frameworks and tools for generative AI development include TensorFlow, PyTorch, and JAX.
Best Practices in Model Development
To ensure successful development of generative AI models, follow these best practices:
1. Iterative Approach: Adopt an iterative approach to model development, allowing for continuous improvement and refinement.
2. Regular Updates: Stay updated with the latest advancements and techniques in generative AI research. Incorporate new methodologies and insights into your model
development process.
3. Collaboration: Foster collaboration between data scientists, domain experts, and stakeholders throughout the development lifecycle. Encourage open communication and knowledge sharing to drive innovation.
4. Ethical Considerations: Consider ethical implications associated with generative AI, such as bias in generated outputs and potential misuse of technology. Implement
safeguards and ethical guidelines to mitigate risks and promote responsible AI development.
By following these guidelines, you can navigate the complexities of generative AI model development effectively and create innovative solutions that drive value for your organization and society as a whole.
Also Read: Leveraging AI In Healthcare Sector
How to apply Generative AI in various industries
Generative AI, a cutting-edge technology, finds diverse applications across various domains, revolutionising how tasks are approached and executed.
Real-World Implementations of Generative AI
Generative AI’s impact is palpable in real-world scenarios, where its capabilities are harnessed to automate and enhance numerous processes. One notable application is code generation, exemplified by Amazon CodeWhisperer, an AI coding companion.
During a productivity challenge, participants leveraging CodeWhisperer experienced a remarkable 27% increase in task completion and accomplished tasks 57% faster than their counterparts who did not use the tool.
Use Cases Across Various Industries
Generative AI transcends industry boundaries, offering solutions tailored to diverse needs. In engineering, it facilitates tasks like intelligent document processing and quality control, while in marketing, it empowers businesses with capabilities such as chatbots and content personalisation.
Moreover, in customer service, generative AI enables the deployment of virtual assistants and intelligent contact centres, enriching the customer experience.
Innovative Applications Driving Technological Advancements
Generative AI serves as a catalyst for technological advancements, fuelling innovation across sectors. Its versatility allows for applications like content creation, where it facilitates the rapid production of diverse creative content, including art, music, animation, and videos.
By leveraging generative AI, businesses can elevate their creative output while optimising resource utilisation.
Popular Generative AI Applications
Generative AI technology has found its way into various domains, revolutionizing industries and sparking innovation across the board. Let’s explore some of the most popular applications where generative AI shines:
1. Image Generation and Manipulation
In the realm of visual content, generative AI has made significant strides, enabling the creation and manipulation of images with unprecedented realism and diversity.
From 3D rendering to avatar creation, generative models empower creators to produce captivating visuals across different mediums.
These models can effortlessly generate intricate graphs displaying novel chemical compounds vital for drug discovery, design logos, enhance existing images, and even produce 3D models tailored for immersive virtual reality experiences.
2. Large Language Models
Language-based generative AI models have unlocked a world of possibilities in textual content creation. Large language models (LLMs) are at the forefront, facilitating tasks such as essay generation, code development, translation, and even comprehension of complex genetic sequences.
With generative AI, the boundaries of linguistic expression and human intelligence are continually pushed, offering solutions to diverse challenges across industries.
3. Natural Language Processing
Natural Language Processing (NLP) stands as a testament to the versatility of generative AI. Through sophisticated algorithms, NLP models interpret and generate human language, enabling applications like sentiment analysis, chatbots, language translation, and content summarization.
With the power of generative AI, language barriers are dismantled, and communication becomes more accessible and efficient.
4. Creative Content Generation
Generative AI fuels creativity by offering novel ways to generate music, audio snippets, and even visual artworks.
By harnessing the capabilities of generative models, creators can develop customized music compositions, recognize objects in videos and synthesize accompanying audio, and generate visual content ranging from illustrations to intricate animations.
These tools empower artists and creators to explore new realms of expression and innovation.
4. Audio and Visual
The fusion of audio and visual elements presents exciting opportunities for generative AI.
Models can seamlessly blend audio and visual components, creating immersive experiences in virtual reality environments, enhancing storytelling in video production, and automating processes such as captioning and image recognition.
With generative AI, the boundaries between auditory and visual mediums blur, ushering in a new era of multimedia creativity.
5. Synthetic Data
Synthetic data generation stands as a cornerstone of modern AI development, addressing data scarcity and quality challenges.
Generative models play a crucial role in creating synthetic data across various modalities, facilitating efficient training of AI models with limited labeled data.
By reducing labeling costs and expanding training datasets, using generative ai AI accelerates the development and deployment of AI solutions across industries.
The applications of generative AI continue to expand, driving innovation and transformation across diverse sectors.
From automotive simulations to healthcare research and entertainment content creation, generative AI is reshaping the way we interact with technology and the world around us.
Also Read: How to Boost Patient Engagement Using Conversational Al.
What are the Benefits of Generative AI?
Generative AI, with its remarkable capabilities, offers a plethora of advantages across various domains, paving the way for innovation and efficiency.
1. Enhancing Creativity and Innovation
Generative AI algorithms empower individuals and industries to unleash their creative potential like never before.
By generating novel content such as images, videos, and text, indistinguishable from human-created content, generative AI fuels creativity in fields like entertainment, advertising, and the arts. This innovation sparks new ideas, fosters artistic expression, and opens doors to groundbreaking possibilities.
2. Improving Efficiency and Productivity
Generative AI algorithms play a pivotal role in enhancing the efficiency and accuracy of existing AI systems. By creating synthetic data, these algorithms enable better training and evaluation of AI models in natural language processing, computer vision, and other domains.
This augmentation leads to more robust and reliable AI systems, ultimately increasing productivity and streamlining processes across industries.
3. Transforming Business Processes
Generative AI’s ability to analyse complex enterprise data and in innovative ways revolutionizes business operations and decision-making processes. By uncovering hidden patterns and trends within vast datasets, businesses and researchers gain valuable insights, driving informed strategies and informed decision-making.
Moreover, the automation and acceleration of various tasks through generative AI reduce manual efforts, saving time and resources while boosting overall efficiency.
Challenges of Generative AI
Generative AI, although promising, faces several significant challenges as it continues to evolve and expand its applications.
These challenges encompass both technical and ethical considerations, requiring careful navigation and innovative solutions to ensure the responsible development and deployment of generative models.
1. Addressing Ethical and Social Implications
One of the foremost challenges in the realm of generative AI revolves around addressing the ethical and social implications of its usage. As generative models become increasingly sophisticated, concerns about their potential misuse and impact on society arise.
Issues such as bias in generated content, misinformation dissemination, and infringement of privacy rights underscore the need for robust ethical frameworks and governance mechanisms.
2. Overcoming Technical Limitations
Technical limitations pose another significant hurdle in the advancement of generative AI. One prominent technical challenge is the scale of compute infrastructure required to train and deploy generative models effectively.
These models often boast billions of parameters and demand vast computational resources, necessitating substantial capital investment and expertise in managing large-scale compute infrastructure for large models.
Moreover, the sampling speed of generative models remains a concern, especially in interactive applications such as chatbots and voice assistants. Delays in generating instances can impede user experience, highlighting the need for optimizations to enhance sampling efficiency and reduce latency.
3. Ensuring Robustness and Reliability
The robustness and reliability of generative AI systems are crucial for their widespread adoption and trust among users. Generative models must demonstrate consistent performance across diverse datasets and scenarios while mitigating the risk of generating inaccurate or misleading outputs.
Additionally, ensuring the security and integrity of generative AI systems against adversarial attacks and manipulation is paramount to safeguarding against potential vulnerabilities and threats.
Despite these challenges, numerous organisations and research institutions are actively
engaged in developing solutions to address the obstacles facing generative AI.
Initiatives aimed at improving data quality, enhancing computational efficiency, and fostering ethical practices are pivotal in shaping the future of generative AI and maximizing its potential benefits while minimising associated risks.
Companies like NVIDIA, Cohere, and Microsoft are at the forefront of innovation in generative AI, offering tools and services designed to alleviate technical complexities and support the responsible deployment of generative models at scale.
By collaboratively addressing these challenges, the generative AI community can pave the way for transformative advancements while upholding ethical standards and societal well-being.
Introducing Voiceoc: Revolutionising Patient Communication
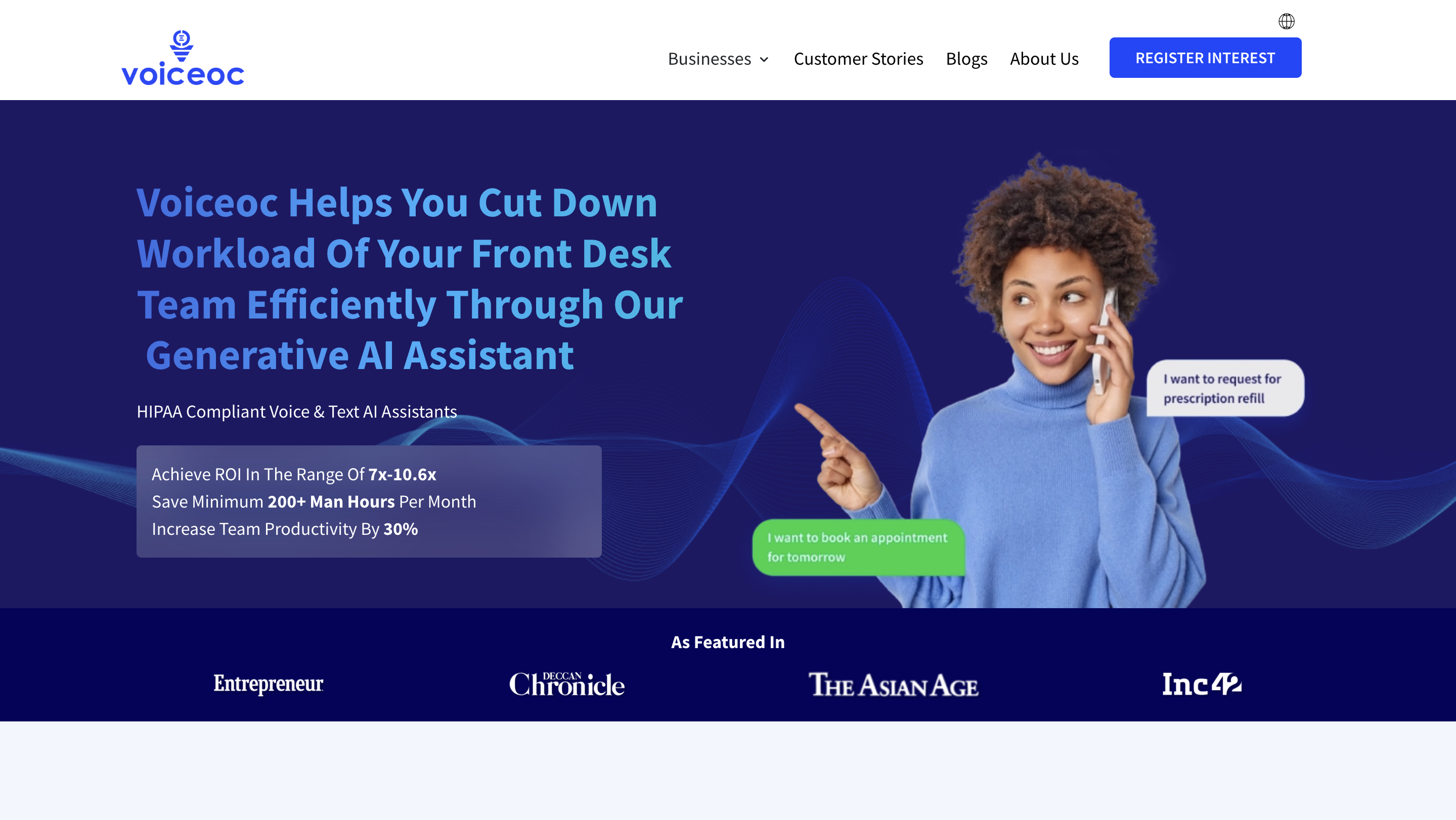
At Voiceoc, we’re dedicated to transforming patient communication with our cutting-edge Voice AI engine. Here’s how our innovative features can enhance your healthcare organisation:
1. Seamless Voice Messaging: Say goodbye to tedious typing! With Voiceoc, patients can effortlessly communicate by sending voice messages, making interactions more natural and convenient.
2. Emotion Tracking: Our AI Sentiment analysis engine monitors patients’ emotions in real-time, allowing you to identify and address any concerns promptly. By converting unhappy patients into satisfied ones, you can foster stronger patient relationships.
3. Agent Assistance: Boost the productivity of your customer care agents with our AI Agent assist module. It understands patients’ needs and efficiently transfers chats to the right agent, ensuring timely support. Track agents’ performance and streamline workflows for enhanced efficiency.
4. Multilingual Support: Break language barriers effortlessly with Voiceoc’s language capabilities. Our AI engine responds in any language, delivering personalised interactions that resonate with your patients, fostering a friendly and inclusive environment.
5. Data-Driven Insights: Leverage patient conversation data to make informed decisions for your organization. Voiceoc provides deep insights into patient engagement, helping you optimize processes, improve patient experience, and outperform competitors. Easily download data for further analysis.
6. Seamless Integration: Voiceoc seamlessly integrates with any HIS/LIS and third-party applications, including payment gateways, CRM systems, and Google APIs. Rest assured that your data remains secure while enjoying the benefits of enhanced connectivity.
Experience the future of patient communication with Voiceoc. Empower your organisation to deliver exceptional care and elevate patient satisfaction to new heights.
Conclusion
Generative AI has emerged as a transformative force across various industries, offering innovative solutions in image generation, text generation, natural language processing, audio and visual manipulation, and synthetic data creation.
Its ability to mimic human creativity and intelligence has opened up new avenues for businesses, researchers, and creators alike.
Looking ahead, the future of generative AI appears promising. As businesses increasingly adopt digital technologies, generative AI is poised to become integral to numerous sectors.
Its applications are expected to expand further, driving advancements in content creation, automation, healthcare, and more. However, the ethical and societal implications of its widespread adoption must be carefully addressed to ensure responsible use and mitigate potential risks.
Voiceoc offers a transformative solution for healthcare organisations seeking to enhance patient engagement and satisfaction.
With innovative features like seamless voice messaging, emotion tracking, and multilingual support, Voiceoc revolutionises patient communication.
By leveraging data-driven insights and seamless integration capabilities, Voiceoc empowers organisations to deliver exceptional care and stay ahead in today’s dynamic healthcare landscape.
Elevate your patient communication with Voiceoc and embark on a journey towards unparalleled patient satisfaction.