Computers are generating original content with a touch of creativity once thought unique to humans. This is the reality with generative AI.
Such systems take inspiration from vast datasets to create something new, whether it’s a piece of music, a novel design, ai algorithms or an innovative code.
You might be wondering, “What’s in it for me?” The advancement of generative AI is a game-changer, regardless of your background. It’s transforming industries and redefining what’s possible with technology.
As we journey through this evolving landscape, it’s vital to stay informed and engage with the technology thoughtfully.
Why is Generative AI important?
Generative AI is revolutionising how we work, with machine learning algorithm automating tasks that traditionally required human intelligence and creativity.
For instance, a machine learning model might design your next marketing campaign, or generative AI could draft software code, speeding up development processes significantly.
Generative AI excels by mimicking human-like creativity. Through advanced techniques and models, it can turn complex data into original, high-quality outputs.
The real magic happens in its application across various industries, from automating routine tasks to pioneering new research and development avenues.
How Do Generative AI Operate?
Ever wonder how generative AI turns data into new creations? It’s less about pulling rabbits out of hats and more about how these systems use data, or training data, to craft something new. It’s a bit like a chef using ingredients to come up with a new recipe.
Generative AI models sift through data, find patterns, and use these insights to generate new content that resembles the original in style but is entirely new.
Generative AI stands out in the AI landscape for its ability to create new, original content from existing data. At the heart of generative AI are machine learning models, which, unlike their traditional counterparts, don’t just interpret data but use it to generate new instances that didn’t exist before.
Traditional machine learning models primarily focused on discrimination, aiming to classify data
points by establishing relationships between known and unknown factors.
For instance, when examining images, these models analyze known data such as pixel arrangements, lines, colors, and shapes, and then correlate them with unknown factors like words.
This process involves identifying mathematical equations that can numerically map these known and unknown factors as variables, typically denoted as x and y.
Generative models take a step beyond this approach. Instead of merely predicting a label given certain features, they strive to predict features given a specific label.
Mathematically, generative modeling calculates the probability of the occurrence of both x and ytogether, learning the distribution of various data features and their interconnections.
For example, generative models scrutinize animal images to discern variables such as different ear shapes, eye shapes, tail features, and skin patterns.
By learning these features and their relationships, they gain an understanding of what different animals generally look like and can subsequently generate new animal images that were not part of the original training set.
Types of Generative AI Models
There are several broad categories of generative AI models:
1. Diffusion Models
These models generate new data by iteratively introducing controlled random changes to an initial data sample. They start with the original data and progressively add subtle changes (noise) to make it less similar to the original.
This noise is carefully regulated to ensure the coherence and realism of the generated data. After several iterations of adding noise, the diffusion model reverses the process, gradually removing the noise to produce a new data sample resembling the original.
2. Generative Adversarial Networks (GANs)
GANs operate by training two neural networks in a competitive manner. The generator network creates fake data samples by incorporating random noise, while the discriminator network attempts to distinguish between real and fake data.
Through iterative training, the generator improves its ability to produce realistic data, while the discriminator becomes better at identifying fake data.
GANs find applications in generating realistic images, style transfer, and data augmentation.
3. Variational Auto Encoders (VAEs)
VAEs learn a compact representation of data known as the latent space. This space represents the data in a mathematical form, akin to a unique code based on all its attributes. VAEs employ two neural networks—the encoder and the decoder. The encoder maps the input data to a mean and variance for each dimension of the latent space, generating a random sample from a Gaussian distribution. The decoder then reconstructs this sampled point from the latent space back into data resembling the original input.
4. Transformer-Based Models
These models build upon the encoder and decoder concepts of VAEs and incorporate self-attention mechanisms and contextual embeddings to process sequences of data efficiently.
Self-attention enables the model to focus on relevant elements within the sequence, while multiple encoder layers known as attention heads learn to attend to different parts of the input sequence simultaneously.
Additionally, each layer refines the contextual embeddings, making them more informative and capturing various aspects from grammar syntax to complex semantic meanings.
The Generative Process: From Data Points to Creative
Content
The magic begins with data points – the raw material for generative AI. Through a combination of unsupervised and semi-supervised deep learning method and techniques, these models sift through and analyse the data, identifying underlying patterns and structures.
This process is not just about replication but about understanding the essence of the content to produce something new that maintains the integrity of the original data.
Take, for example, the task of image generation. A generative AI model studies numerous images, learning about shapes, colors, and textures, and then uses this knowledge to create a completely new image that resembles real-life objects but is entirely original.
Similarly, in text generation, the model predicts the next word in a sentence based on the context provided by the preceding words, gradually building coherent and meaningful paragraphs.
One of the breakthroughs in generative AI is the development of models like Stable Diffusion, which can generate photorealistic and realistic images just from textual descriptions, and GPT-3, which can produce essays, code, or even poetry based on brief prompts.
These advancements underscore the versatility and creative potential of generative AI, making it a powerful tool for innovation across various fields.
As generative AI systems continue to evolve, they have become indispensable tools in various domains, including business processes and creative content generation.
Leveraging sophisticated algorithms and vast datasets, these systems can analyse complex data, identify patterns, and generate valuable insights. Additionally, generative AI work enable the creation of realistic images, text, and multimedia content, revolutionising content creation workflows.
By harnessing the power of generative AI, businesses can streamline operations, enhance productivity, and unlock new opportunities for innovation and growth.
How will generative AI enhance business value?
Generative AI offers fresh and transformative opportunities to increase revenue, reduce costs, improve productivity, and enhance risk management. In the foreseeable future, it is poised to confer a competitive edge and serve as a distinguishing factor.
Revenue Enhancement:
1. Product Innovation: Generative AI empowers enterprises to expedite the development of innovative products. These could span from new pharmaceuticals,
environmentally-friendly household cleaners, unique flavours and fragrances, innovative alloys, to swifter and more accurate diagnoses.
2. Diversified Revenue Streams: Enterprises with advanced AI capabilities are projected to reap greater revenue benefits, as indicated by Gartner’s research.
Cost Reduction and Productivity Enhancement:
1. Augmentation of Workforce: Generative AI has the potential to enhance workers’ capacity to compose and refine textual content, images, and multimedia. It can also
streamline content summarisation, simplification, and classification; generate, translate, and validate software code; and enhance chatbot efficiency. Currently, the technology excels in swiftly generating a wide array of artefacts at scale.
2. Long-Term Talent Optimisation: Employees will be characterised by their adeptness in conceptualising, executing, and refining ideas, projects, processes, services, and
relationships alongside AI. This collaborative synergy will expedite skill acquisition and significantly broaden the capabilities of the workforce across various domains.
3. Process Refinement: Generative AI has the potential to extract tangible, contextually-relevant insights from extensive repositories of content, thus revolutionising workflows.
Risk Management Opportunities:
1. Risk Mitigation: Generative AI’s prowess in analyzing and offering comprehensive insights into data, such as customer transactions and potentially flawed software code,
enhances pattern recognition and facilitates quicker identification of potential risks to the enterprise.
2. Sustainability: Generative AI has the potential to support enterprises in compliance with sustainability regulations, reducing the risk of stranded assets, and incorporating
sustainability considerations into decision-making, product design, and operational processes.
The Impact and Utility of Generative AI
Transforming Industries: Practical Uses of Deep Generative Models
Applications
Many generative ai models are reshaping industries by introducing efficiency and innovation.
- For instance, in engineering, generative AI models such as diffusion models assist in creating complex designs, streamlining the design process significantly and generating realistic images.
- Similarly, in the pharmaceutical industry, these models accelerate drug discovery by predicting new chemical compounds, thereby speeding up the research and
development phase. - For content creation, generative AI is a versatile tool capable of producing diverse forms of content, from written articles to music and images. This not only aids in content and image generation but also opens up new avenues for creative expression.
- Healthcare benefits from generative AI through the creation of synthetic data, which enhances research capabilities while maintaining patient confidentiality.
How Generative AI is Shaping the Future
Generative artificial intelligence is revolutionising how we approach problem-solving and creativity, offering a spectrum of benefits that were once considered the realm of science fiction.
- For instance, in the world of content creation, generative AI models automate and enhance the writing process, turning a blank page into a draft filled with potential,
thereby streamlining workflows significantly. - Moreover, the technology extends its advantages to complex data analysis, where it interprets and simplifies information, making it more accessible.
- Imagine generative AI systems analyzing vast amounts of data to predict trends in business or healthcare, thus enabling proactive decision-making.
- One of the most compelling uses of generative AI is in training machine learning models where training data might be scarce or sensitive.
- By generating synthetic data, these AI systems can fill in gaps, ensuring that machine learning models are both robust and privacy-compliant.
What are the Limitations of Generative AI systems?
Despite its vast potential, the generative AI model is not without its challenges and ethical considerations.
- As these models become more adept at analysing complex data and generating realistic content, the line between real and AI-generated content blurs, raising concerns about misinformation and the authenticity of digital content.
- One of the primary challenges is ensuring that the generative AI models are free from biases present in the training data. Given that these models learn from existing datasets, there’s a risk of perpetuating stereotypes or biases if the training data isn’t carefully curated.
- Moreover, the issue of data privacy comes to the forefront, especially when generative AI models are trained on sensitive or personal information.
- Ensuring that these systems respect user privacy and adhere to data protection regulations is paramount.
Overview of Generative AI Tools and AI Systems
Generative artificial intelligence is not just a concept; it’s a rapidly evolving field with tools and technologies that drive innovation across various sectors.
At the core of these advancements are generative AI models, which differ from traditional machine learning models by their ability to generate new data points rather than just classifying existing ones.
For businesses and creators, this translates into a suite of generative AI tools that can automate content creation, enhance design processes, and even aid in decision-making.
These tools are powered by sophisticated AI systems, including diffusion models and neural networks, which are the backbone of creativity and innovation in the generative AI landscape.
One notable application is in content creation, where generative AI models are used to produce written content, visual art, and even music, mimicking human creativity but at a scale and speed unattainable by humans alone.
In the realm of business, these tools are revolutionizing workflows by automating routine tasks, thus freeing up human talent to do multiple tasks and focus on more complex and creative endeavours.
From Diffusion Models to Neural Networks: The Engine Behind Creativity
The magic behind generative AI’s capability to create lies in its underlying models and algorithms. Diffusion models, for example, start with a dataset and iteratively introduce small, controlled changes until new, realistic data samples are generated.
This process is akin to an artist starting with a rough sketch and gradually refining it into a detailed painting.
Neural networks, particularly deep learning models, are another cornerstone of generative AI. They analyze vast datasets to learn patterns and features, which they then use to generate new data that shares similarities with the original set.
For instance, after studying numerous images of animals, a generative AI model can produce entirely new images of animals that look realistic but do not replicate any specific animal that exists.
Generative AI vs. Traditional AI: Understanding the differences
Generative artificial intelligence represents a frontier in AI technology that diverges significantly from traditional AI.
While traditional AI focuses on analyzing and making decisions based on existing data,
generative AI takes a leap forward by creating new data that didn’t exist before.
This could be anything from novel artwork and music to synthetic data sets and even realistic chat responses.
At the heart of generative AI are advanced neural network techniques such as Generative Adversarial Networks (GANs), Variational Autoencoders (VAEs), and Transformer models.
These models enable generative AI to produce highly original content. In contrast, traditional AI might employ Convolutional Neural Networks (CNNs) for image recognition tasks or Recurrent Neural Networks (RNNs) for sequence analysis, focusing more on interpreting existing data rather than creating anew.
One of the key differentiators is how generative AI begins with a basic prompt or input, using it as a springboard to generate a wide array of outputs.
This stands in contrast to traditional AI systems that typically process data through a more rigid, rule-based approach, leading to a predictable outcome.
Generative AI vs. Predictive and Conversational AI
Generative AI sets itself apart not only from traditional models but also from predictive and conversational AI. Predictive AI, as the name suggests, specialises in forecasting future events based on historical data.
It’s a powerful tool for businesses looking to glean actionable insights from past trends and patterns. Generative AI, while also capable of leveraging data, is more focused on the creation of new content or data that mimics the original in a novel way.
Conversational AI, on the other hand, is designed to facilitate seamless interactions between humans and machines. It relies heavily on natural language processing (NLP) and machine learning to understand and respond to human language in a natural, human-like manner.
Generative AI contributes to this field by enabling more dynamic, contextually relevant responses that can greatly enhance the user experience.
Natural language processing (NLP) is at the core of generative AI, facilitating the understanding and generation of human language.
Powered by sophisticated algorithms, NLP enables generative AI systems to parse, interpret, and generate text with remarkable fluency and coherence.
These language models, a subset of generative AI, leverage NLP techniques to generate content ranging from articles and stories to chat responses and poetry.
With advancements in NLP, generative AI tools have become increasingly adept at understanding context, nuances, and linguistic intricacies, ushering in a new era of human-machine interaction and collaboration.
Also Read: How to Boost Patient Engagement Using Conversational Al
What are the Applications of Generative AI?
Generative artificial intelligence is transforming industries with its ability to create novel content, designs, and solutions.
Here are some of the most prevalent applications of generative AI:
1. Language: Text serves as the foundation for numerous generative AI models and is recognized as one of the most advanced domains. Large language models (LLMs) represent a prominent example within this domain, deployed for a diverse array of tasks such as essay generation, code development, translation, and comprehension of genetic sequences.
2. Audio: The realms of music, audio, and speech are burgeoning fields within generative AI. Applications include the development of songs and audio snippets from text inputs, object recognition in videos with corresponding audio effects, and custom music composition.
3. Visual: Generative AI finds extensive application in image-related tasks, including the generation of 3D images, avatars, videos, graphs, and other visualizations.
These models offer versatility in producing images with varied aesthetic styles and feature techniques for editing and altering generated visuals.
They contribute to diverse applications such as generating chemical compound graphs for drug discovery, creating lifelike images for virtual and augmented reality, designing video game assets, crafting logos, and enhancing or modifying existing images.
4. Synthetic Data: Synthetic data generation plays a pivotal role in training AI models, particularly when authentic data is scarce, restricted, or insufficient to address diverse scenarios accurately.
Generative models facilitate the development of synthetic data through label-efficient learning techniques, thereby mitigating labeling costs and enhancing AI model training with augmented or internally learned data representations.
Also Read: Leveraging AI In Healthcare Sector
What are the impact of Generative AI Models on different
industries?
The impact of generative models extends across various domains, with applications expanding continuously. Some examples include:
● Automotive Industry: Generative AI is poised to aid in the creation of 3D environments and models for simulations and vehicle development. Synthetic data is utilized for
training autonomous vehicles, enabling realistic road testing to enhance safety, efficiency, and flexibility while reducing risks and overhead costs.
● Natural Sciences: Generative AI contributes significantly to medical research by generating new protein sequences for drug discovery. It automates tasks such as
medical scribing, coding, imaging, and genomic analysis in healthcare.
● In weather forecasting, user generative ai models simulate planetary conditions, facilitating accurate predictions and preparations for natural disasters, thereby enhancing public safety.
● Entertainment Industry: From video games to film production, animation, world-building, and virtual reality, all facets of the entertainment industry leverage generative AI models to streamline content creation.
These models serve as tools to supplement creativity and workflow efficiency for creators across various media platforms.
How to Stay Informed and Engaged with Generative AI Developments?
Staying ahead in the fast-evolving field of generative AI requires a proactive approach. For those looking to dive deeper, engaging with a plethora of online courses ranging from beginner to advanced levels can provide a solid foundation in understanding and leveraging generative AI technologies.
These courses often cover the essentials of neural networks, diffusion models, and more, tailored for both developers and business professionals.
Moreover, joining online communities and forums dedicated to AI research can offer valuable insights and discussions on the latest developments in generative AI.
These platforms are treasure troves of knowledge, where enthusiasts and experts alike share resources, tutorials, and personal experiences with generative AI tools and applications.
For professionals looking to integrate generative AI into their workflows, attending webinars and conferences can provide not only the latest knowledge but also networking opportunities with pioneers in the field.
These events often showcase real-world applications of generative AI, offering a glimpse into the future of various industries.
Voiceoc: Empowering Healthcare with Generative Artificial Intelligence
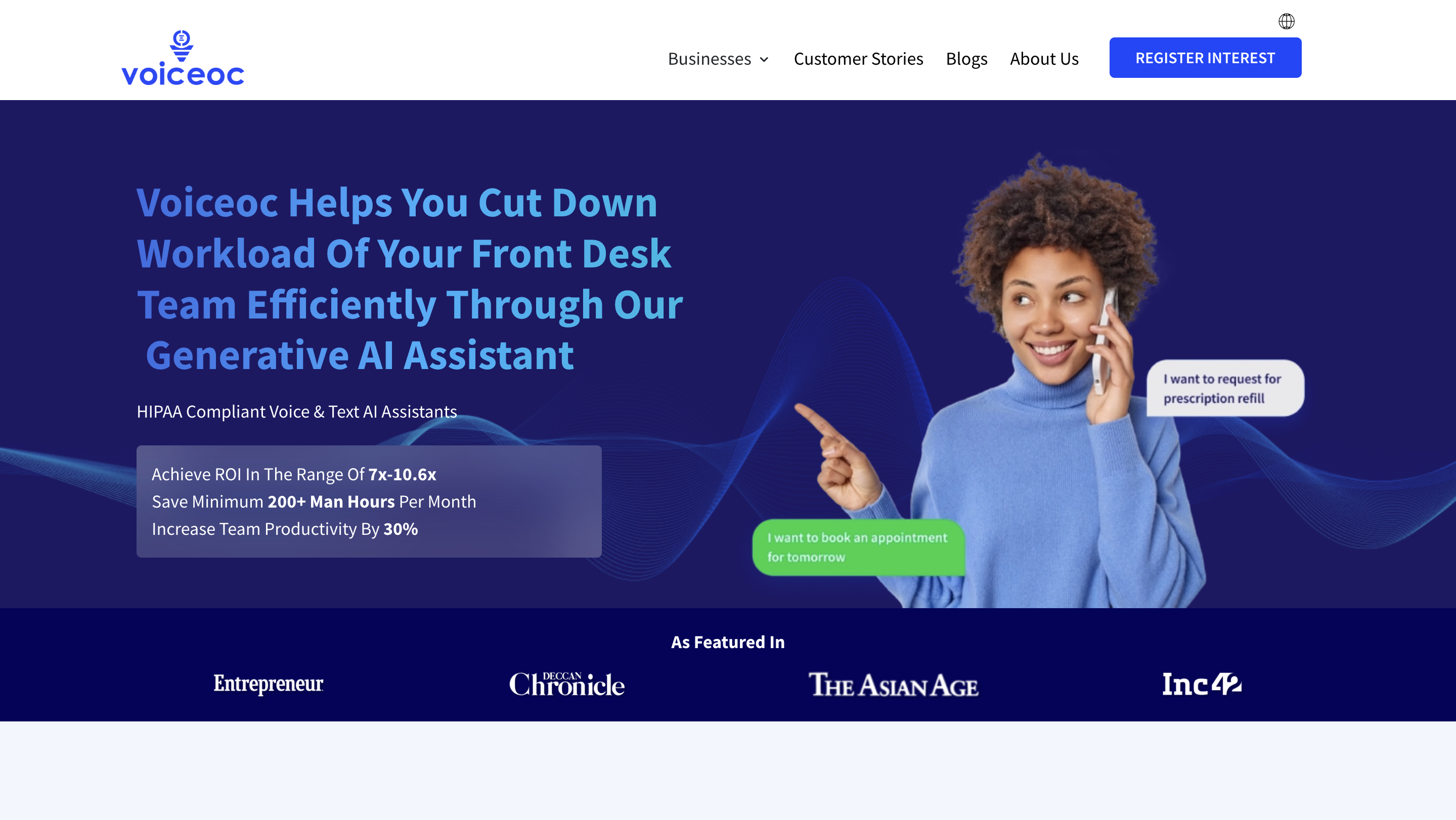
Voiceoc brings a seamless solution to the forefront, enhancing patient experience and streamlining administrative tasks within healthcare facilities.
1. Appointment Management
Voiceoc streamlines the appointment booking process, empowering patients to manage their appointments conveniently.
Whether integrated with Electronic Health Records (EHR) systems or operating independently, Voiceoc ensures flexibility and efficiency in scheduling.
2. On-Demand Support
With Voiceoc, patients can receive immediate assistance without the need for human intervention.
By delivering personalized responses to inquiries regarding billing, doctor availability, navigation, and insurance, Voiceoc enhances patient satisfaction and reduces administrative burden.
3. Patient-Reported Outcomes
Voiceoc facilitates proactive healthcare management by enabling the collection of patient-reported outcomes.
By regularly checking on patient health and advising relevant action steps, Voiceoc fosters continuous engagement and encourages follow-up appointments, ultimately leading to better health outcomes.
4. Regular Updates
Voiceoc keeps patients informed and engaged by providing regular updates and reminders.
Whether it’s appointment reminders, video teleconsultation links, or feedback prompts, Voiceoc ensures that patients feel supported and cared for throughout their healthcare journey, thereby strengthening the patient-provider relationship.
Conclusion
The interplay between generative ai and human ingenuity marks a pivotal chapter in our technological journey. Generative AI, with its ability to craft everything from intricate art pieces to complex musical compositions, is not just a tool; it’s a collaborator that amplifies human creativity.
The synergy of human oversight combined with the generative modeling prowess of AI models like GPT-3 and Stable Diffusion is birthing unprecedented innovations across sectors.
In the creative industries, artists are leveraging generative AI to push the boundaries of their craft, creating pieces that meld human emotion with AI precision.
In the business realm, generative AI systems are streamlining workflows, from automating routine tasks to using enterprise data and generating insightful data analyses, thereby freeing up human talent for strategic thinking and innovation.
The road ahead promises even greater collaboration, where generative AI becomes an integral part of our daily tools, enhancing everything from writing aids to design software. This integration will make advanced AI capabilities accessible to all, democratising creativity and innovation.
Key Points to Remember
● Ethical Use: As we integrate AI into more aspects of life, understanding and addressing ethical implications is crucial.
● Business Application: Generative AI isn’t just about creativity; it’s a tool for enhancing efficiency and innovation in businesses.
● Technological Understanding: Knowing how generative AI works, from neural networks to machine learning models, empowers us to use it responsibly and effectively.
In the realm of artificial intelligence, large language models have emerged as transformative tools. These models, trained on vast amounts of data, exhibit remarkable capabilities in understanding and generating human-like text.
Among them, generative models stand out for their ability to create new content autonomously, opening up possibilities across various domains. Within this landscape, machine learning models play a pivotal role, serving as the backbone for training and refining generative AI systems.
These machine learning models undergo extensive training on diverse datasets, enabling them to learn complex patterns and generate novel outputs.
As the field progresses, the synergy between generative AI systems and machine learning models continues to drive innovation and advancement.
Voiceoc, empowered by generative artificial intelligence, transforms healthcare delivery by seamlessly integrating cutting-edge technology with patient-centric care. With Voiceoc, healthcare providers can revolutionize patient engagement, optimize administrative workflows, and deliver personalized care experiences.
By harnessing the power of generative AI, Voiceoc ensures enhanced efficiency, improved patient outcomes, and a brighter future for healthcare delivery.
Join the journey towards innovation and excellence with Voiceoc, where generative AI meets healthcare excellence.